Exciting Problem in Cardiology -- Machine Learning Diagnosis of Irregular Heartbeats
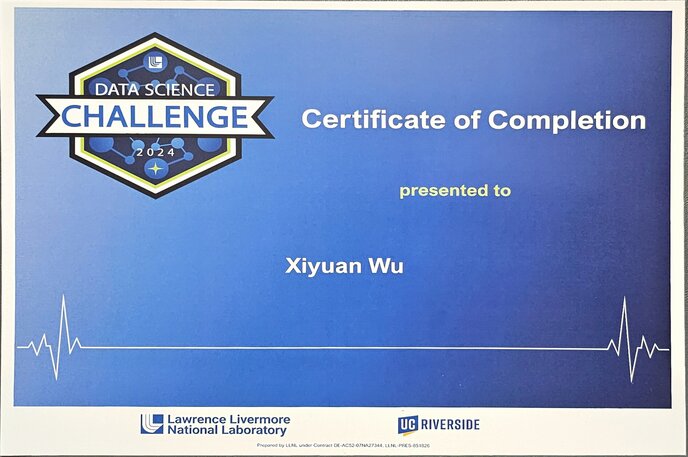
The experience of this challenge was deeply impactful. I hope this is not just an end, but a new beginning. In the future, many more challenges await me!
Abstract & Background
Arrhythmia is a medical condition characterized by irregular heartbeats, which, if left untreated, can have life-threatening effects. This project leverages machine learning to detect heart abnormalities using inexpensive electrocardiogram (ECG) data. Our model can classify ECG data as usual or one of four abnormal heartbeats with a false negative rate of only 0.87%. Additionally, our model's predictions for myocardial activation times are, on average, only 2 ms off. These results suggest that machine learning is a promising, cost-effective solution for arrhythmia detection.
Heart disease is the leading cause of death in the United States. Arrhythmias are a result of underlying heart problems and classifying them can provide life-saving care. A common method of getting heartbeat data is a standard 12 lead ECG, where electric signals from the heart are measured. We aim to use these signals to predict when specific parts of the heart activate.